As I was texting my friends about a birthday gift for someone, I replied: “Dat is vast een leuke verassing.” [That’s probably a nice cremation]. For non-Dutch speakers, this might sound shocking, but I wasn’t actually talking about cremation. The Dutch words for ‘surprise’ and ‘cremation’ are “verrassing” and “verassing,” respectively. My friends, all Dutch language students, found this hilarious, and I didn’t hear the end of it for weeks. However, this mistake got me thinking: why does autocorrect fix spelling but can’t understand the meaning of what I’m saying? Why can’t it prevent these kinds of semantic errors?
A few weeks later, in the same chat group, someone mixed up “peddelen” with “padellen” [paddling vs. playing padel], which led to more teasing but also deepened my curiosity. Why does autocorrect, which can suggest words that match my sentence well, fail to correct these semantically illogical sentences? Why can’t it help with meaning as well as spelling? And how might this be improved in messaging apps or word processors like Word?
What is autocorrect?
Autocorrect is software that automatically corrects spelling and typing errors across various programs and apps. Its main goal is to help users type faster and with fewer mistakes by fixing common errors, such as misspellings or swapped letters (e.g., changing “teh” to “the”). While it is easy to take for granted today, autocorrect has evolved over decades.
The roots of autocorrect go back to the early 1990s when Microsoft engineer Dean Hachamovitch worked on autocorrect for Microsoft Word. In its earliest form, it was a simple tool designed to improve typing speed by replacing common typos with their correct forms. It focused on word substitution and didn’t account for sentence context or structure. Over time, it developed to fix capitalization errors and homophones (like “their” vs. “there”), but still didn’t understand the meaning behind sentences.
With the rise of mobile technology, autocorrect shifted to more advanced algorithms that analyze context, keyboard proximity, and usage patterns. These systems allow users to type quickly on small touchscreens, as Wired noted:
Without it, we probably couldn’t even have phones that look anything like the ingots we tickle—the whole notion of touchscreen typing, where our podgy physical fingers are expected to land with precision on tiny virtual keys, is viable only when we have some serious software to tidy up after us.
The limits of autocorrect
Despite these advancements, autocorrect still has its limitations. It can fix spelling errors and offer word suggestions based on context, but it doesn’t always grasp the meaning of what you’re writing. This is where things can go wrong, as in my “cremation” example. Although autocorrect uses complex algorithms to predict and correct based on patterns, its focus is primarily on spelling and grammar, leaving a gap in understanding meaning.
Why didn’t autocorrect realize I was talking about a birthday gift, and that ‘cremation’ was completely out of place? Or why couldn’t it detect that my friend’s mix-up between ‘paddling’ and ‘padel’ didn’t fit the context of our conversation? The issue, I believe, is that today’s autocorrect systems lack a deep understanding of semantics—the actual meanings behind the words.
Why isn’t context enough?
To explain why autocorrect isn’t flawless, I have to make some educated guesses based on my studies in linguistics. First, the size of the dataset used to train autocorrect plays a big role. Languages with larger speaker communities naturally generate more data, which can be used to train more accurate algorithms. The accuracy of autocorrect may correlate directly with the availability of this data.
Second, the homogeneity of a language affects autocorrects success. Dialects, slang, and loanwords vary by region and time, so what texts are chosen to train the algorithm heavily influences its accuracy.
Third, sentence specificity matters. If you type “I’m going to the stor,” autocorrect easily assumes you meant “store.” However, if you type something like “I’m thinking about going to a computer stor,” it may struggle since the phrase is less common in training data.
The answer: AI?
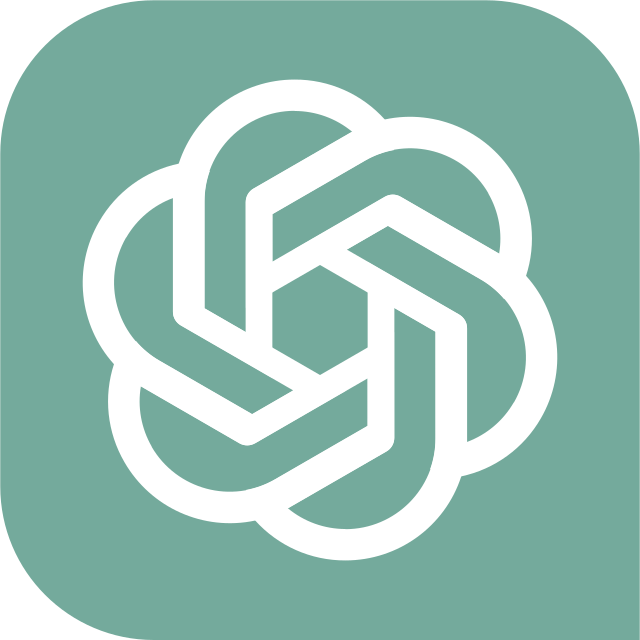
Natural Language Processing (NLP) models, such as ChatGPT, have shown a greater ability to understand the intended meaning of a sentence compared to traditional autocorrect algorithms. This difference comes down to the type of coding: autocorrect algorithms are largely rule-based, whereas NLPs use machine learning, which allows them to detect and process semantic patterns more effectively. Another key factor is data size; NLPs are trained on vast, diverse datasets, enabling them to analyse both syntax and meaning in context, offering more accurate suggestions than traditional autocorrect systems.
Conclusion
Autocorrect has evolved significantly, but it still falls short when it comes to understanding the deeper meaning of our messages. While traditional systems excel at correcting spelling and grammar, they lack the semantic insight to catch errors like confusing “cremation” with “surprise.” As AI and NLP technology continue to develop, integrating these more advanced models into autocorrect systems could bridge this gap, allowing for not just correct spelling but also logical, contextually accurate communication.
Very entertaining read – I haven’t really thought of the history behind autocorrect, but I have in fact wondered why it seems so delayed in advancement compared to other types of technology. Sometimes when typing on my laptop I catch myself making (what seems like) a common typo and rely on autocorrect to fix it for me, yet it quite often leaves me disappointed. Also one thing I have noticed by the people around me is that whenever someone get a new phone, one of the first actions to take is to get rid of the autocorrect on it. It very often corrects words which don’t even need correction, but the system assumes you meant to write something else (reference to the meme where someone wanted to write “hows life babygirl”, but it got autocorrected into “hows life baby gorilla”).
At the same time I am addmitedly writing this comment in Word to make sure any typos get flagged and corrected (in contrast to me immediately writing it as a comment on the site, where it doesn’t point to any mistakes). Therefore its safe to conclude that autocorrect holds a very love-hate relationships whith most people since it can be useful when typing longer forms of text, but often proves itself as annoying when you are casually texting friends.
I think your proposed solution makes loads of sense and can actually turn autocorrect in a loved and useful tool in all contexts.
I just noticed a typo in my comment – “whith” instead ot “with” – so turns out even me typing it in Word coulnd save me.
I can totally relate to this story because my name is Jet Lurvink, but autocorrect always changes it to Het Lurvink, no matter how many times I have written my name! It is so frustrating that it doesn’t learn something so personal and obvious over time. I really liked how the blog combined your studies with this course. That said, I also wonder if relying on autocorrect too much could make us lazy since we don’t even have to type out full words anymore…