Just have a look at the following picture:
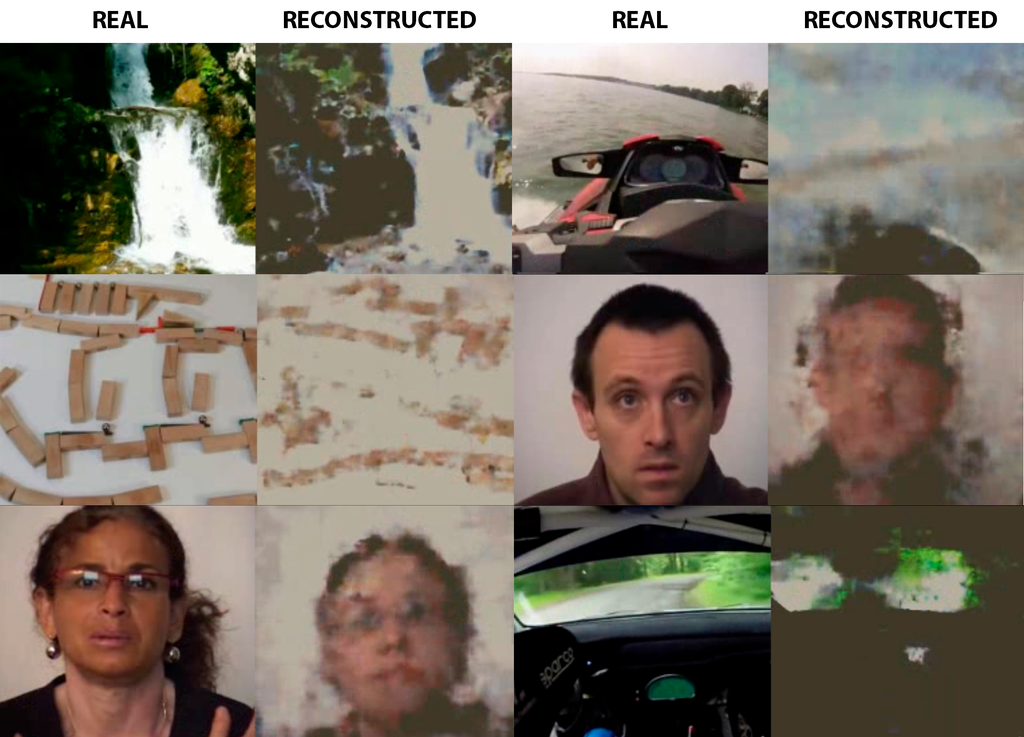
What do you think is happening here?
One might think that the pictures depicted show how an Artificial Intelligence (AI) is taking in “real” pictures and subsequently tries to draw its “own version” of the original image.
Is it an AI applying a filter to distort the image? Did the AI see the original picture at all?
Reconstructing human thoughts in real time
If you have not noticed until now: Yes, we do live in the future. What authors have depicted in science fiction novels just a few years ago is happening right now!
Because what you are actually seeing is an AI reconstructing an image, merely from the brain waves recorded by an EEG.
The researchers from the Russian corporation Neurobotics and the Moscow Institute of Physics and Technology have discovered a way to construct an actual image visualization out of the data from an electroencephalography (EEG) in real time.
Basically, the researchers are measuring brainwaves with an EEG and feed it to a neural network that will try to recreate the image the participants are seeing live in form of a short YouTube clip.
“We’re working on the Assistive Technologies project of Neuronet of the National Technology Initiative, which focuses on the brain-computer interface that enables post-stroke patients to control an exoskeleton arm for neurorehabilitation purposes, or paralyzed patients to drive an electric wheelchair, for example. The ultimate goal is to increase the accuracy of neural control for healthy individuals, too.”
Vladimir Konyshev, head of the Neurorobotics Lab
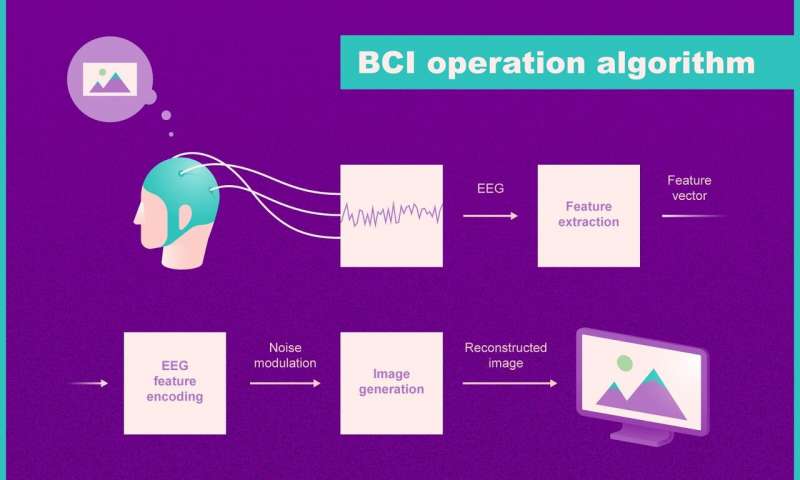
The experiment was structures as follows: The researchers asked healthy participants to watch 20 minutes video material consisting of 10-second YouTube video clips. These clips were in either one of 5 arbitrary video categories: abstract shapes, waterfalls, human faces, moving mechanisms and motor sports.
Analyzing the EEG data, the researchers were able to show, that the subjects brain wave patterns are distinct for each video category. If the participants was shown a video containing human faces, the brain wave patterns are similar across different clips that also contain human faces.
After this discovery was made, the scientists were able to develop a neural network that could be trained with EEG data. Having trained the network, the AI was capable of turning EEG signals into actual images similar to the videos the subjects were viewing.
“The electroencephalogram is a collection of brain signals recorded from scalp. Researchers used to think that studying brain processes via EEG is like figuring out the internal structure of a steam engine by analyzing the smoke left behind by a steam train. We did not expect that it contains sufficient information to even partially reconstruct an image observed by a person. Yet it turned out to be quite possible.”
Grigory Rashkov, co-author & junior researcher at MIPT
And it can only improve from here…
If scientists are already able to reconstruct the brain imaginary of a human in a somewhat “readable” visualization, then what can be expected out of this technology in the future?
While the authors of this article are mainly focused on providing tools that enhance the quality of live for stroke-patients, it would probably not be too far off to assume that this technology could possibly used for recording dream-imagery or enhancing visual fidelity of people with obstructed vision.
Finding out how the brain is encoding visual imagery could also lead to further advancements in medicine, especially in curing people with strong vision impairments or blindness.
Just as expected, Artificial Intelligence networks are and will be able to aid our research in ways that have been previously unimaginable. With newly learned helpers, who knows what we can and will discover about our brain and how far we can go down the rabbit hole of technological advancements and augmentations that will help the impaired and increase general quality and ease of life.
Those images of comparison actually have quite accurate results, which is fascinating! Do you happen to know if the reconstructed image is created in real-time like a video? Or does it have to render for a while before constructing?
The article says that the AI constructed video would be in real-time!
hey leon,
Very interesting topic. I didn’t know that this is already happening. Scary and fascinating at the same time. In future there won`t be secrets anymore haha