Social media studies have occupied quite a significant amount of scholarly discussion in the fields of communication, political science and other social sciences alike. Though with the advancement of the discipline, some issues remain unsolved.
What to study and how to study them?
The essential question to ask is then what social media studies intend to study and what methodologies can we utilise to achieve that. The following table provides a general overview of the field, partially inspired by Wietske Van Osch and Coursaris (2015) and Berger (2019):
Topics |
Education and Learning |
Culture and Community |
Healthcare |
Demographics |
Career |
Politics |
Psychological well-being |
Theories |
Cooperation Theory |
Network Theory |
Social Exchange Theory |
Social Capital Theory |
Social Identity Theory |
Theory of Planned Behaviours and Theory of Reasoned Action |
Technology Acceptance Model |
Methods |
(Automated) Quantitative Content Analysis |
Qualitative Content Analysis: Discourse Analysis, Narrative Analysis and Rhetoric Analysis |
Online Experiment and Survey |
Digital Ethnography |
Interviews and Focus groups |
Data Donation and Mock Environment
Two notable recent developments of methods of studies are data donation and mock environment. The underlying framework of data donation is analogous to the mechanism of data tracking of web browsers. In 2021, a collective of Dutch universities formed a consortium to establish a Digital Data Donation Infrastructure (D3I) , a tool that aims to “provide the legal and methodological framework necessary to help accelerate and expand critical Social Sciences & Humanities research across universities and disciplinary boundaries.” By the form of data donation, researchers can avoid the problems that are generated by the typical shortcomings of lab and online experiments and surveys. Data Donations have a benefit for the donors as well: It enhances the awareness about algorithmic surveillance throughout the period of research. Nonetheless, data donation is confined by participation bias. Who are willing to partake the research and install a browser extension to be monitored for academic purposes? Conceivably, the participants are potentially more aware of the subject matter, or at least more technologically abled.
Another latest methodological advancement is the introduction of mock environments. The goal of mock environments is to maximise the ecological validity of research results, in which users can interact with other users and/or bots in real-time within a mock social media environment. Though mock environments offer a promising foundation for researchers, it is not without limitations. Users are too accustomed to platforms such as Facebook, Twitter and Reddit and the GUIs of these platforms are simply ingrained in our auto-pilots systems. A slight modification of such the graphical environment will change people’s behaviours, notwithstanding the observer effects that come along with that. Recalling a conversation with one of the developers in the PRODIGI team from UZH, another major challenge of a mock environment is the inability to conduct studies with a longer timespan. In other words, observations are limited to only a short period of time (e.g., one hour). Meanwhile it may be able to test certain hypotheses, researchers may not be able to capture long-term effects of repeated exposure and engagement with users and content on social media.
Bypassing consent: Studying Instant Messaging (IM) platforms
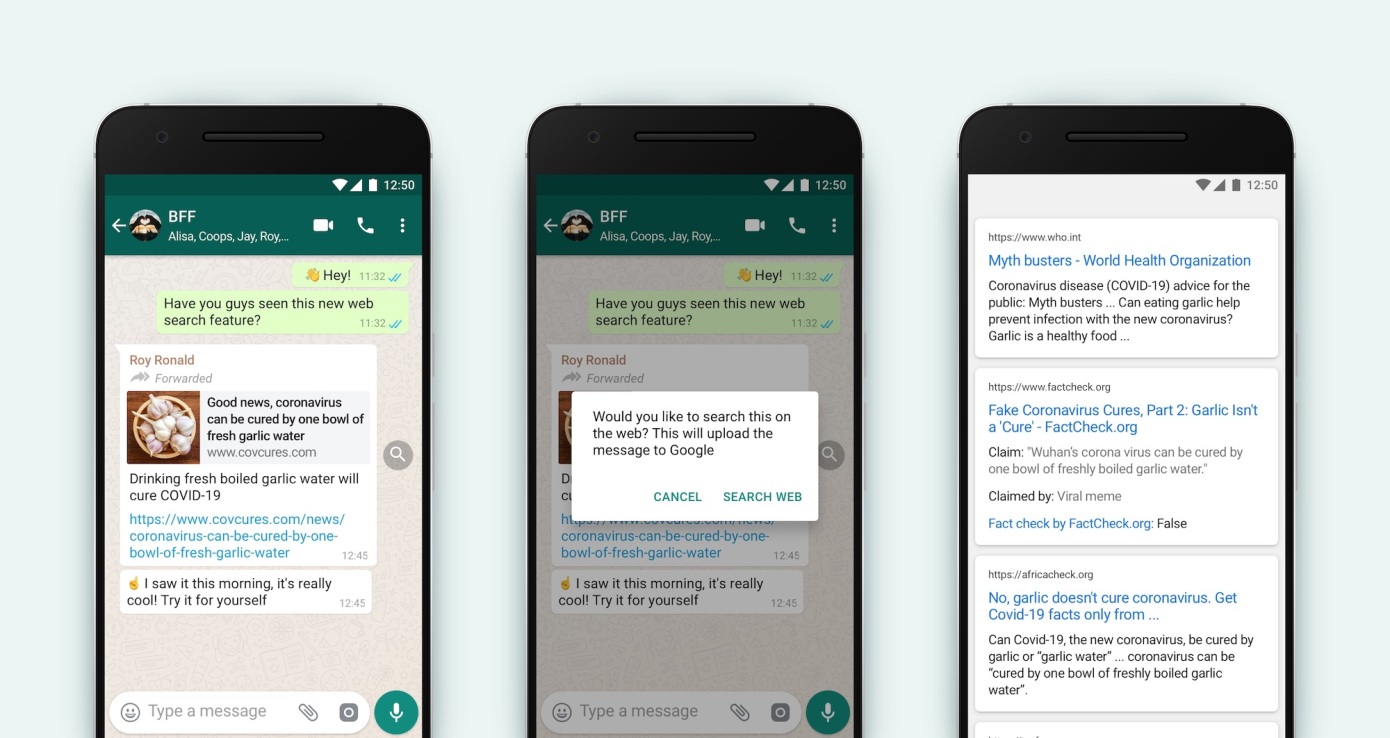
How about studying Instant Messaging platforms like WhatsApp, Telegram and Signal? Given the private nature of IM platforms, it turned out studying IM platforms are even more daunting than open platform, which research are facilitated and encouraged by the platforms themselves. Although IM platforms are less studied than open social media platforms, the effects of interpersonal and at times one-to-many messages (e.g., Telegram channel with a few admins and many followers) on our society must not be ignored. For instance, the POLARCHATS project initiated by the researchers at Carlos III – Juan March Institute (UC3M) reflected the power of misinformation and disinformation that are spread through interpersonal messaging softwares.
As aforementioned, due to the private nature of IM platforms and the content itself, researchers cannot simply hop in the chat and start collecting data like how they do with Twitter and Facebook using their public APIs. It then poses a set of issues to researchers who would like to conduct research on IM platforms. The most pressing issue, though, is that the consent must be sought before the start of data collection. Researchers thus often find themselves in an ethical conundrum. What if one user opt out for the research, can they still collect data from a channel with more than 1000 people? Or in another case, what if new joiners reject to partake in the study, is it a responsibility of the channel administrator to shut the new joiners off from the channel for the sake of academic studies? These questions are rather legally intricate but at the same time a core normative challenge that researchers must overcome with comprehensive solutions.
Dilemma between automated and manual methods
Faced with rising economic and environmental costs, the deep-learning community will need to find ways to increase performance without causing computing demands to go through the roof. If they don’t, progress will stagnate. We must either adapt how we do deep learning or face a future of much slower progress.
Neil C. Thompson, Kristjan Greenewald, Keehon Lee & Gabriel F. Manson on Deep Learning’s Diminishing Returns
Ultimately, we ought not to neglect the dilemma between the use of automated and manual methods. Social media studies, in particular those using computational methods, rely much on large-scale machine learning and deep-learning models that allow for highly functioning and accurate automated content analyses. Communication scholars have previously compared the performance of trained human coders, crowdsourcing, dictionaries and machine learning approaches in sentiment analysis tasks, they discovered that although the three machine learning models (i.e., CNN, SVM and NB) generally provided better accuracy than off-the-shelf dictionaries, the gaps are marginal and fall far behind trained human coders and crowdsourcing coders.

State-of-the-art machine learning models opened new doors for researchers, yet performance improvement requires extreme amount of energy and resources. Both Natural Language Processing (NLP) and Computer Vision are popular methods used among communication scholars, however, the training and improvement of these state-of-the-art models come with immense environmental and economic costs. Take ImageNet for example, if we are to improve error rate from 9% to 5%, the projected increase of environmental costs using a polynomial model will raise from 108 to 1016 lbs of CO2. Now, we are standing at the crossroad of the diminishing marginal returns of deep learning algorithms that may slow down the application and efficiencies of the methods it originally heralded.
The prospects of social media studies
To end with an optimistic note, these challenges are not insurmountable as suggested by the scholars who encountered them. In spite of the obstacles, for example the opaqueness of platforms like TikTok and the unexplored areas such as cross-device comparison, researchers and institutions are dedicating much resource to uncover the benefits and downfalls of social media.
Recent Comments