Last week, I came across a post which highlights one of the issues of training AI with massive data sets and little oversight.
Discussed is a translation by Google Translate from Finnish — a language without gendered pronouns — to English. Now Translate has to choose the gender, and there comes the trouble.
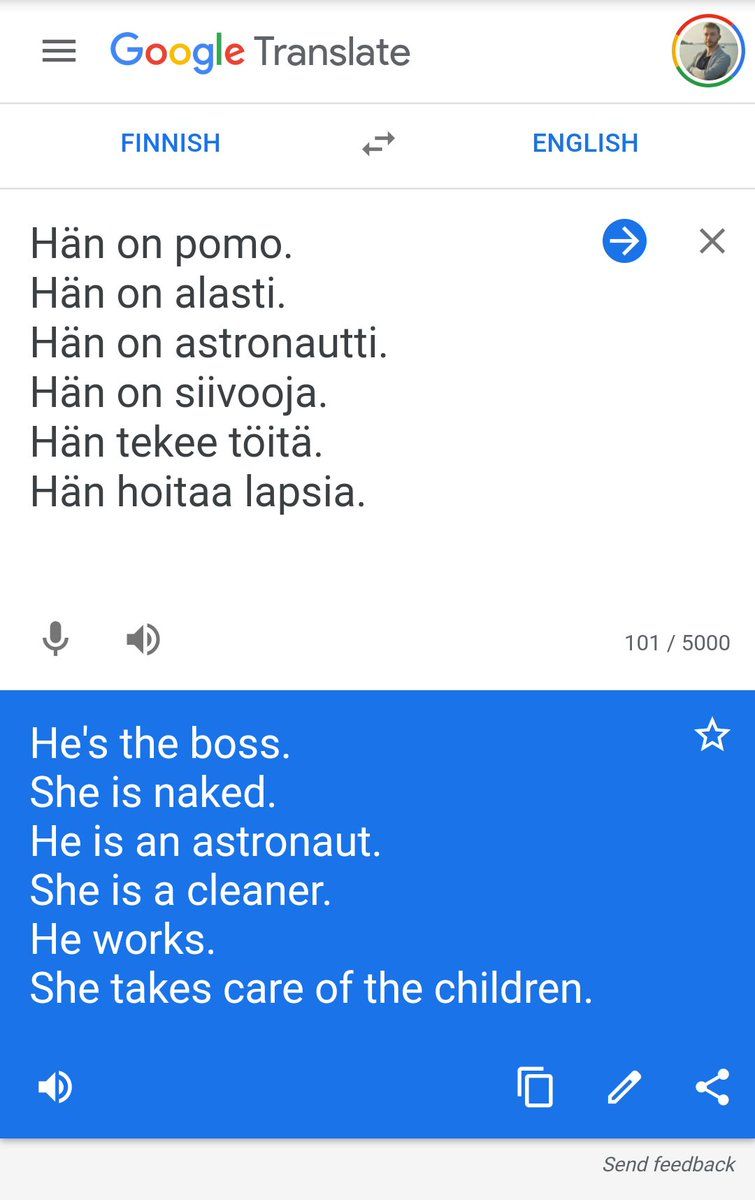
So why the associations of ‘boss’ with ‘man’, and ‘naked’ with ‘woman’?
Google Translate uses an AI trained with data found on the web. When translating a sentence such as ‘the congressman gave his speech‘, the translator can pick up context such as the gendered job title and male pronoun to pick the right gender. However, if there are no such context clues, the translator picks the translation that is statistically more likely to be correct, based on the training data.
Excuses
I want to highlight some of the responses to the post about ‘responsibility’ in this situation.
Algorithms like this are trained end-to-end. They are designed to read a massive text dataset, and learn from it by going over what is said by people on internet, and they do it perfectly. If you want to change their output, change the society
Arash M.
Changing society is quite the task! This is not something the developers of the translator can do easily. But these biased translations can have a negative impact on society, by propagating stereotypes.
[…] The complexity of a service like google translate is immense, and some mistakes and misinterpretations like these take place eventually, especially between genderless and non-genderless languages. This is a little bit forced and unnecessary given the time and hassles these apps save us. […]
Iustin G.
Mistakes happen. But we should not ignore them because they don’t matter all that much in a translation service. If a translator can be biased, other services can be as well. Women can be disadvantaged by AI recruiting tools, hurting their chances of getting jobs. And algorithms for predicting liver disease are wrong twice as often when applied on women.
Maybe a bias in translation is not the most urgent problem, but it highlights issues AI tools have with biases.
Responsibility
So whose responsibility is it to make sure translations aren’t biased towards some gender? There are three points at which this bias can be challenged.
First there is the user. The fact is that some translations cannot be perfect, because there is a disconnect in gender between the two languages. Even if the translator is not biased one way or another, it can only guess at what the user means and therefore get it wrong. There is no ambiguity if the user specifies the gender they mean.
Then there is the training data. The translator is biased, as we’ve seen in the example. If the data used to train the translator is not biased, it would also not give biased translations. But training an AI requires massive amounts of data. The only way to feasibly get this is to crawl the web for it. Because the web is a reflection of our society, existing biases will always appear.
Finally, if the data can’t be fixed, developers of AI can offer alternative ways of overcoming bias. In the case of the translations, Translate now notifies you when sentences contain gender-specific alternatives.
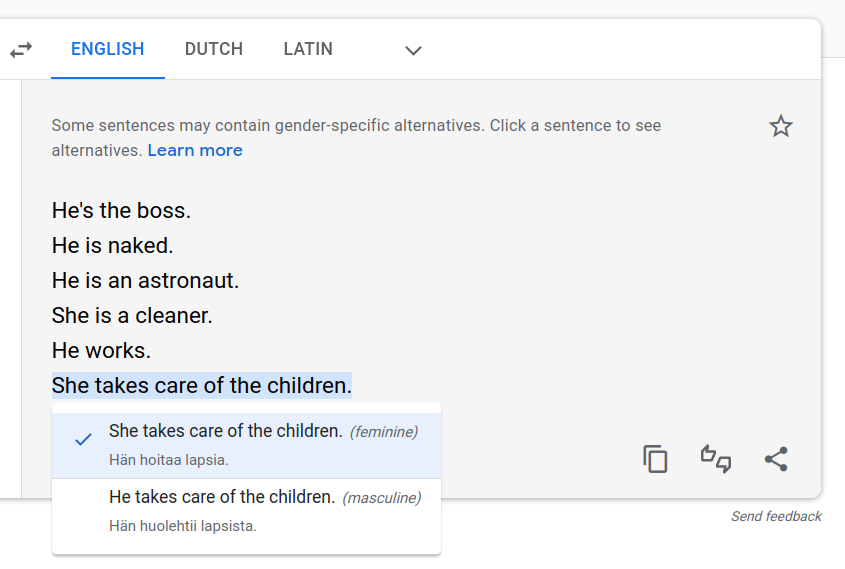
Good! But if you look at the defaults shown, you’ll see that we’re not quite there yet!
Oh wow, I did not know that Finnish is a language without gendered pronouns! How interesting to see how Google gives its own interpretation to genderless pronouns when translating to English. I found this very interesting to read, because it’s something that I have never really thought about. Sad to see that it’s something that cannot be fixed really easily…
Very interesting post! I almost couldn’t believe the translated results, they are that ridiculous. I hope and wish problems like this were more discussed and taken seriously, since they do pile up and result in actual discrepancies and although humans cannot really ever escape their biases we can strive to make technology as unbias as possible.